Model-Based Imitation Learning for Urban Driving
Anthony Hu Gianluca Corrado Nicolas Griffiths Zak Murez Corina GurauHudson Yeo Alex Kendall Roberto Cipolla Jamie Shotton
Wayve, University of Cambridge
Paper Blog Code
An accurate model of the environment and the dynamic agents acting in it offers great potential for improving motion planning. We present MILE: a Model-based Imitation LEarning approach to jointly learn a model of the world and a policy for autonomous driving. Our method leverages 3D geometry as an inductive bias and learns a highly compact latent space directly from high-resolution videos of expert demonstrations. Our model is trained on an offline corpus of urban driving data, without any online interaction with the environment. MILE improves upon prior state-of-the-art by 31% in driving score on the CARLA simulator when deployed in a completely new town and new weather conditions. Our model can predict diverse and plausible states and actions, that can be interpretably decoded to bird’s-eye view semantic segmentation. Further, we demonstrate that it can execute complex driving manoeuvres from plans entirely predicted in imagination. Our approach is the first camera-only method that models static scene, dynamic scene, and ego-behaviour in an urban driving environment.
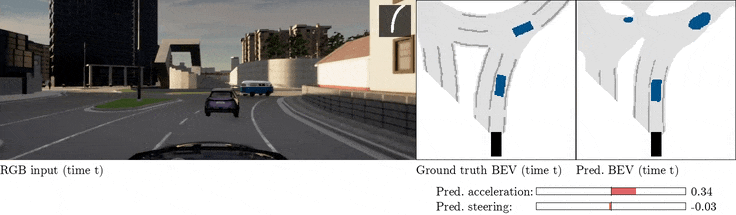
From left to right we visualise: RGB input, ground truth bird's-eye view semantic segmentation, predicted bird's-eye view segmentation.
When the RGB input becomes sepia-coloured, the model is driving in imagination.